
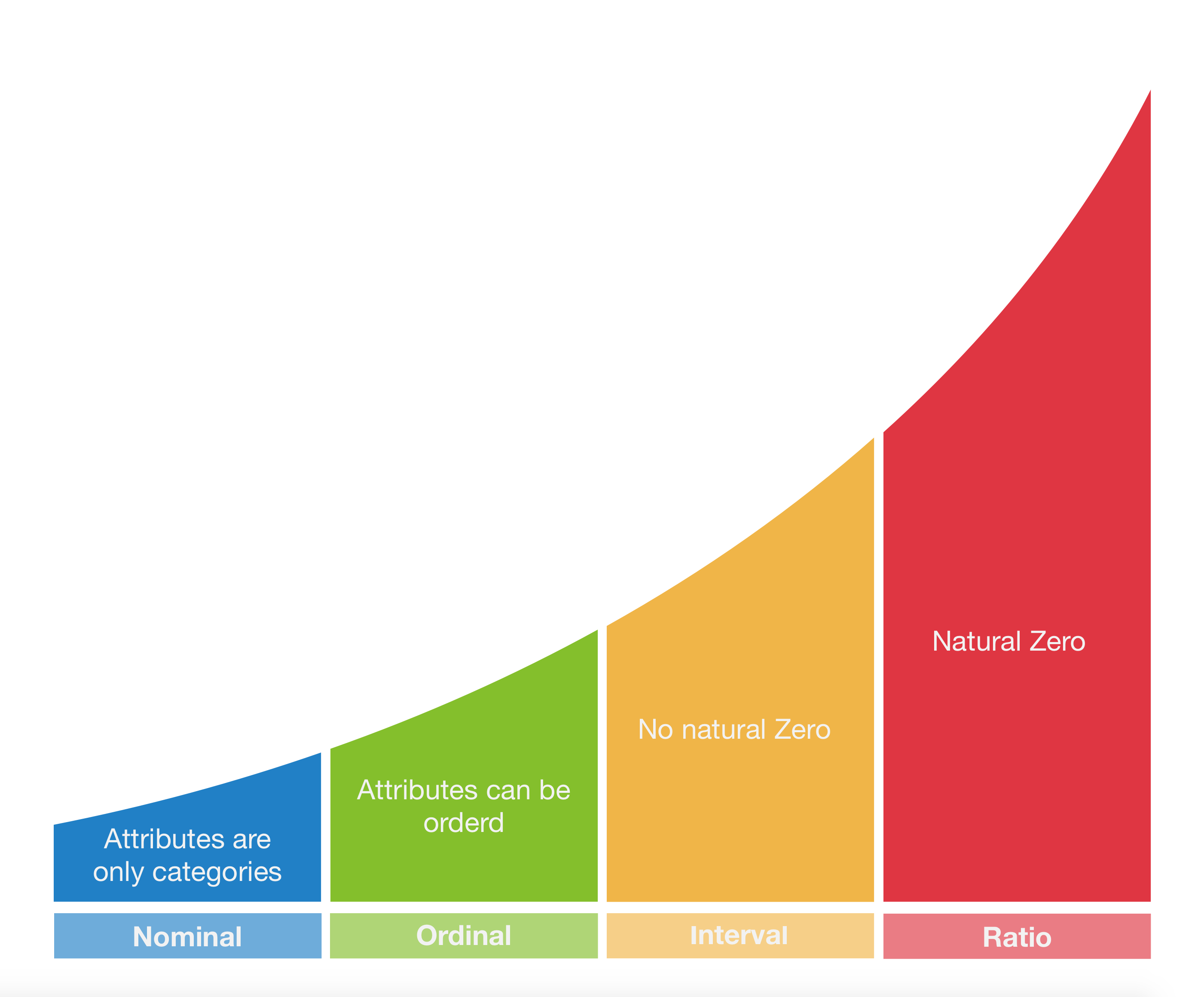
The machine learning algorithms learn from data, identify patterns and make decisions. Machine learning is a method of data analysis that learns information directly from data to automate analytical model building. It has been widely accepted as the standard tool for describing and comparing the accuracy of diagnostic tests.įor example, you can use ROC Curve analysis to test a diagnostic to determine if an incident had occurred, or compare the accuracy of two methods that are used to discriminate diseased cases versus healthy cases. ROC (Receiver Operating Characteristic) curve analysis is mainly used for diagnostic studies in Clinical Chemistry, Pharmacology and Physiology. It can compute the power of the experiment for a given sample size, and can also compute the required sample size for given power values. Power and Sample Size analysis is useful for researchers to design their experiments. We suppose that the survival function follows a Weibull distribution and fit the model with a maximum likelihood estimation. Weibull fit is a parameter method to analyze the relationship between the survival function and the failure time. It relates the time of an event, usually death or failure, to a number of explanatory variables known as covariates. The proportional hazards model, also called Cox model, is a classical semi-parameter method.

In addition to estimating the survival functions, Kaplan-Meier Estimator in Origin provides three other methods to compare the survival function between two samples: Kaplan-Meier Estimator, a non-parametric estimator, uses product-limit methods to estimate the survival function from lifetime data. It often reveals relationships that were previously unsuspected, thereby allowing interpretations of the data that may not ordinarily result from examination of the data. PLS can be used to discover important features of a large data set. PLS is most commonly used for constructing predictive model when the information contained in a large number of original variables and they are highly collinear. There are two primary reasons for using PLS: Partial Least Squares regression (PLS) is used for constructing predictive models when there are many highly collinear factors.
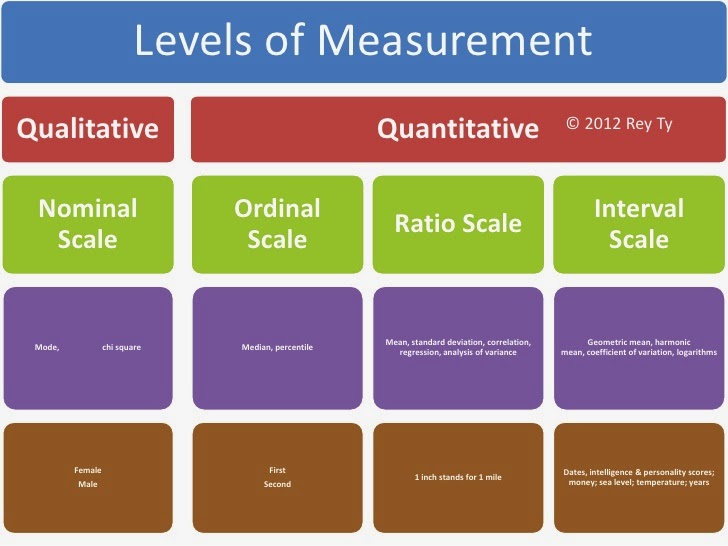
It is faster than Hierarchical but need user know the centroid of the observations, or at least the number of groups to be clustered.ĭiscriminant analysis is used to distinguish distinct sets of observations, and to allocate new observations to previously defined groups. Use K-means clustering to classify observations through K number of clusters.
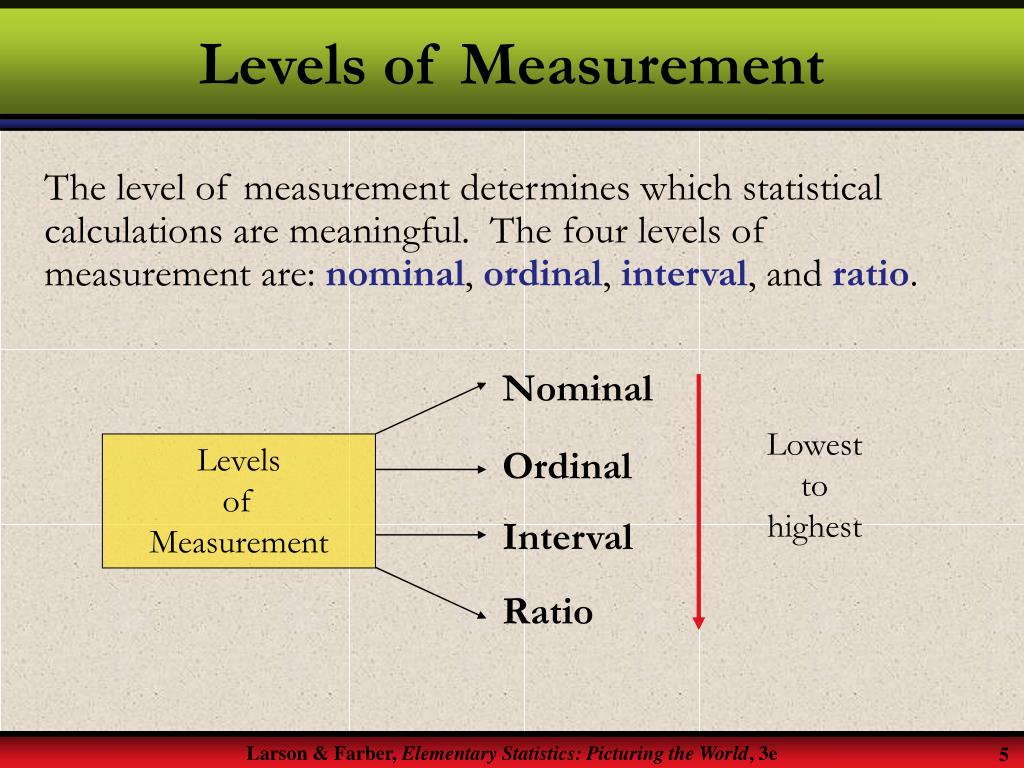
In this method, elements are grouped into successively larger clusters by some measures of similarity or distance. This form of analysis is an effective way to discover relationships within a large number of variables or observations. PCA is thus often used as a technique for reducing dimensionality.Ĭluster analysis is used to construct smaller groups with similar properties from a large set of heterogeneous data. Principal Component Analysis (PCA) is used to explain the variance-covariance structure of a set of variables through linear combinations of those variables.
